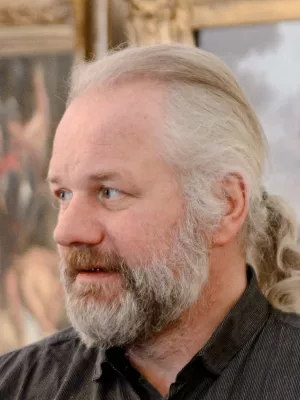
Leif Lönnblad
Professor
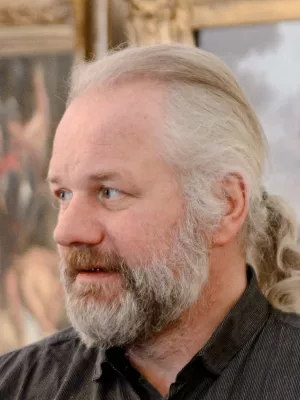
Self-organizing networks for extracting jet features
Author
Summary, in English
Self-organizing neural networks are briefly reviewed and compared with supervised learning algorithms like back-propagation. The power of self-organization networks is in their capability of displaying typical features in a transparent manner. This is successfully demonstrated with two applications from hadronic jet physics; hadronization model discrimination and separation of b, c and light quarks.
Department/s
- Department of Astronomy and Theoretical Physics - Has been reorganised
Publishing year
1991-12
Language
English
Pages
193-209
Publication/Series
Computer Physics Communications
Volume
67
Issue
2
Document type
Journal article
Publisher
Elsevier
Topic
- Subatomic Physics
Status
Published
ISBN/ISSN/Other
- ISSN: 0010-4655