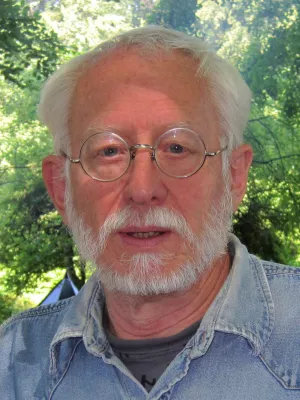
Lars Gislén
Retired, affiliated researcher
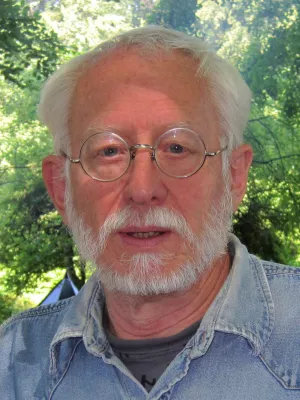
Complex Scheduling with Potts Neural Networks
Author
Summary, in English
In a recent paper (Gislén et al. 1989) a convenient encoding and an efficient mean field algorithm for solving scheduling problems using a Potts neural network was developed and numerically explored on simplified and synthetic problems. In this work the approach is extended to realistic applications both with respect to problem complexity and size. This extension requires among other things the interaction of Potts neurons with different number of components. We analyze the corresponding linearized mean field equations with respect to estimating the phase transition temperature. Also a brief comparison with the linear programming approach is given. Testbeds consisting of generated problems within the Swedish high school system are solved efficiently with high quality solutions as results.
Department/s
- Computational Biology and Biological Physics - Has been reorganised
Publishing year
1992
Language
English
Pages
805-831
Publication/Series
Neural Computation
Volume
4
Issue
6
Document type
Journal article
Publisher
MIT Press
Topic
- Computer and Information Science
Status
Published
ISBN/ISSN/Other
- ISSN: 1530-888X